Supported Indicators
Mesa Adaptive Moving Average
Introduction
Implements the Mesa Adaptive Moving Average (MAMA) indicator along with the following FAMA (Following Adaptive Moving Average) as a secondary indicator. The MAMA adjusts its smoothing factor based on the market's volatility, making it more adaptive than a simple moving average.
To view the implementation of this indicator, see the LEAN GitHub repository.
Using MAMA Indicator
To create an automatic indicators for MesaAdaptiveMovingAverage
, call the MAMA
helper method from the QCAlgorithm
class. The MAMA
method creates a MesaAdaptiveMovingAverage
object, hooks it up for automatic updates, and returns it so you can used it in your algorithm. In most cases, you should call the helper method in the initialize
method.
class MesaAdaptiveMovingAverageAlgorithm(QCAlgorithm): def initialize(self) -> None: self._symbol = self.add_equity("SPY", Resolution.DAILY).symbol self._mama = self.mama(self._symbol, 0.5, 0.05) def on_data(self, slice: Slice) -> None: if self._mama.is_ready: # The current value of self._mama is represented by self._mama.current.value self.plot("MesaAdaptiveMovingAverage", "mama", self._mama.current.value) # Plot all attributes of self._mama self.plot("MesaAdaptiveMovingAverage", "fama", self._mama.fama.current.value)
The following reference table describes the MAMA
method:
mama(symbol, fast_limit=0.5, slow_limit=0.05, resolution=None, selector=None)
[source]Creates a new Mesa Adaptive Moving Average (MAMA) indicator. The MAMA adjusts its smoothing factor based on the market's volatility, making it more adaptive than a simple moving average.
- symbol (Symbol) — The symbol for which the MAMA indicator is being created.
- fast_limit (float, optional) — The fast limit for the adaptive moving average.
- slow_limit (float, optional) — The slow limit for the adaptive moving average.
- resolution (Resolution, optional) — The resolution
- selector (Callable[IBaseData, IBaseDataBar], optional) — Optional function to select a value from the BaseData. Defaults to casting the input to a TradeBar.
The Mesa Adaptive Moving Average (MAMA) indicator for the requested symbol with the specified limits.
If you don't provide a resolution, it defaults to the security resolution. If you provide a resolution, it must be greater than or equal to the resolution of the security. For instance, if you subscribe to hourly data for a security, you should update its indicator with data that spans 1 hour or longer.
For more information about the selector argument, see Alternative Price Fields.
For more information about plotting indicators, see Plotting Indicators.
You can manually create a MesaAdaptiveMovingAverage
indicator, so it doesn't automatically update. Manual indicators let you update their values with any data you choose.
Updating your indicator manually enables you to control when the indicator is updated and what data you use to update it. To manually update the indicator, call the update
method with a TradeBar
or QuoteBar
. The indicator will only be ready after you prime it with enough data.
class MesaAdaptiveMovingAverageAlgorithm(QCAlgorithm): def initialize(self) -> None: self._symbol = self.add_equity("SPY", Resolution.DAILY).symbol self._mama = MesaAdaptiveMovingAverage(0.5, 0.05) def on_data(self, slice: Slice) -> None: bar = slice.bars.get(self._symbol) if bar: self._mama.update(bar) if self._mama.is_ready: # The current value of self._mama is represented by self._mama.current.value self.plot("MesaAdaptiveMovingAverage", "mama", self._mama.current.value) # Plot all attributes of self._mama self.plot("MesaAdaptiveMovingAverage", "fama", self._mama.fama.current.value)
To register a manual indicator for automatic updates with the security data, call the register_indicator
method.
class MesaAdaptiveMovingAverageAlgorithm(QCAlgorithm): def initialize(self) -> None: self._symbol = self.add_equity("SPY", Resolution.DAILY).symbol self._mama = MesaAdaptiveMovingAverage(0.5, 0.05) self.register_indicator(self._symbol, self._mama, Resolution.DAILY) def on_data(self, slice: Slice) -> None: if self._mama.is_ready: # The current value of self._mama is represented by self._mama.current.value self.plot("MesaAdaptiveMovingAverage", "mama", self._mama.current.value) # Plot all attributes of self._mama self.plot("MesaAdaptiveMovingAverage", "fama", self._mama.fama.current.value)
The following reference table describes the MesaAdaptiveMovingAverage
constructor:
MesaAdaptiveMovingAverage
Implements the Mesa Adaptive Moving Average (MAMA) indicator along with the following FAMA (Following Adaptive Moving Average) as a secondary indicator. The MAMA adjusts its smoothing factor based on the market's volatility, making it more adaptive than a simple moving average.
get_enumerator()
Returns an enumerator that iterates through the history window.
IEnumerator[IndicatorDataPoint]
reset()
Resets the indicator's state, clearing history and resetting internal values.
to_detailed_string()
Provides a more detailed string of this indicator in the form of {Name} - {Value}
str
update(time, value)
Updates the state of this indicator with the given value and returns true if this indicator is ready, false otherwise
- time (datetime)
- value (float)
bool
update(input)
Updates the state of this indicator with the given value and returns true if this indicator is ready, false otherwise
- input (IBaseData)
bool
consolidators
The data consolidators associated with this indicator if any
The data consolidators associated with this indicator if any
ISet[IDataConsolidator]
current
Gets the current state of this indicator. If the state has not been updated then the time on the value will equal DateTime.MinValue.
Gets the current state of this indicator. If the state has not been updated then the time on the value will equal DateTime.MinValue.
IndicatorDataPoint
fama
Gets the FAMA (Following Adaptive Moving Average) indicator value.
Gets the FAMA (Following Adaptive Moving Average) indicator value.
IndicatorBase[IndicatorDataPoint]
is_ready
Returns whether the indicator has enough data to be used (ready to calculate values).
Returns whether the indicator has enough data to be used (ready to calculate values).
bool
item
Indexes the history windows, where index 0 is the most recent indicator value. If index is greater or equal than the current count, it returns null. If the index is greater or equal than the window size, it returns null and resizes the windows to i + 1.
Indexes the history windows, where index 0 is the most recent indicator value. If index is greater or equal than the current count, it returns null. If the index is greater or equal than the window size, it returns null and resizes the windows to i + 1.
IndicatorDataPoint
name
Gets a name for this indicator
Gets a name for this indicator
str
previous
Gets the previous state of this indicator. If the state has not been updated then the time on the value will equal DateTime.MinValue.
Gets the previous state of this indicator. If the state has not been updated then the time on the value will equal DateTime.MinValue.
IndicatorDataPoint
samples
Gets the number of samples processed by this indicator
Gets the number of samples processed by this indicator
int
warm_up_period
Gets the number of periods required for warming up the indicator. 33 periods are sufficient for the MAMA to provide stable and accurate results,
Gets the number of periods required for warming up the indicator. 33 periods are sufficient for the MAMA to provide stable and accurate results,
int
window
A rolling window keeping a history of the indicator values of a given period
A rolling window keeping a history of the indicator values of a given period
RollingWindow[IndicatorDataPoint]
Visualization
The following image shows plot values of selected properties of MesaAdaptiveMovingAverage
using the plotly library.
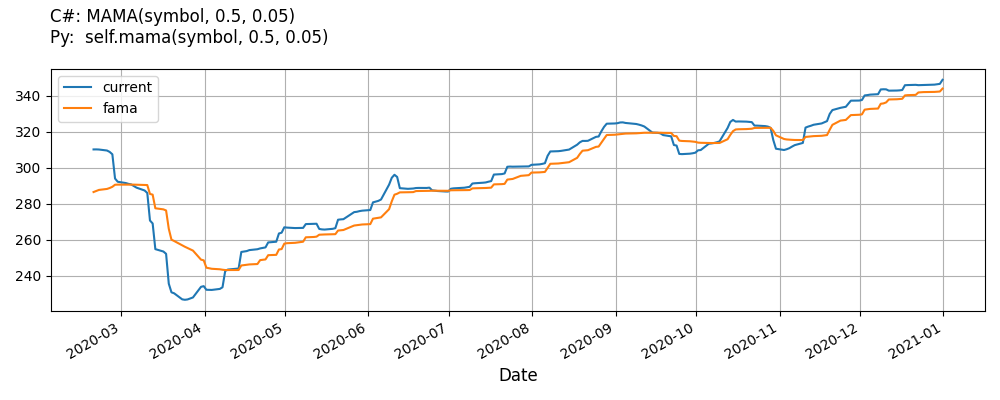
Indicator History
To get the historical data of the MesaAdaptiveMovingAverage
indicator, call the self.indicator_history
method.
This method resets your indicator, makes a history request, and updates the indicator with the historical data.
Just like with regular history requests, the indicator_history
method supports time periods based on a trailing number of bars, a trailing period of time, or a defined period of time.
If you don't provide a resolution
argument, it defaults to match the resolution of the security subscription.
class MesaAdaptiveMovingAverageAlgorithm(QCAlgorithm): def initialize(self) -> None: self._symbol = self.add_equity("SPY", Resolution.DAILY).symbol mama = self.mama(self._symbol, 0.5, 0.05) count_indicator_history = self.indicator_history(mama, self._symbol, 100, Resolution.MINUTE) timedelta_indicator_history = self.indicator_history(mama, self._symbol, timedelta(days=10), Resolution.MINUTE) time_period_indicator_history = self.indicator_history(mama, self._symbol, datetime(2024, 7, 1), datetime(2024, 7, 5), Resolution.MINUTE)
To make the indicator_history
method update the indicator with an alternative price field instead of the close (or mid-price) of each bar, pass a selector
argument.
indicator_history = self.indicator_history(mama, 100, Resolution.MINUTE, lambda bar: bar.high) indicator_history_df = indicator_history.data_frame
To access the properties of the indicator history, index the DataFrame with the property name.
fama = indicator_history_df["fama"] # Alternative way # fama = indicator_history_df.fama