Popular Libraries
Tensorflow
Create Subscriptions
In the Initialize
initialize
method, subscribe to some data so you can train the tensorflow
model and make predictions.
self._symbol = self.add_equity("SPY", Resolution.DAILY).symbol
Build Models
In this example, build a neural-network regression prediction model that uses the following features and labels:
Data Category | Description |
---|---|
Features | The last 5 close price differencing compared to current price |
Labels | The following day's price change |
The following image shows the time difference between the features and labels:
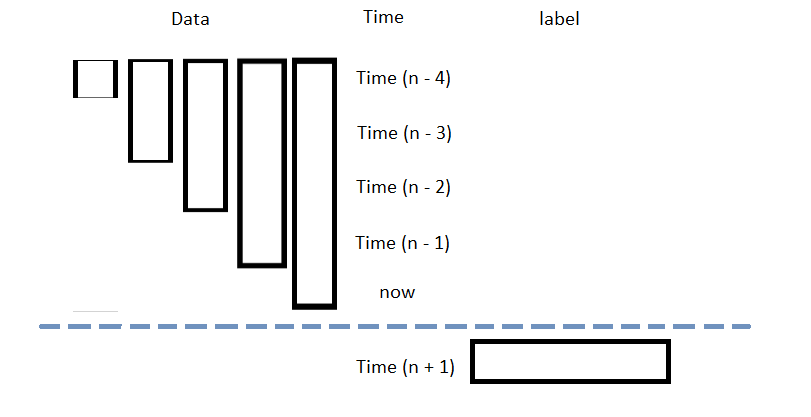
Follow these steps to create a method to build the model:
- Define some parameters, including the number of factors, neurons, and epochs.
- Create the model using built-in Keras API.
num_factors = 5 num_neurons_1 = 10 num_neurons_2 = 10 num_neurons_3 = 5 self.epochs = 20 self.learning_rate = 0.0001
self.model = tf.keras.sequential([ tf.keras.layers.dense(num_neurons_1, activation=tf.nn.relu, input_shape=(num_factors,)), # input shape required tf.keras.layers.dense(num_neurons_2, activation=tf.nn.relu), tf.keras.layers.dense(num_neurons_3, activation=tf.nn.relu), tf.keras.layers.dense(1) ])
Train Models
You can train the model at the beginning of your algorithm and you can periodically re-train it as the algorithm executes.
Warm Up Training Data
You need historical data to initially train the model at the start of your algorithm. To get the initial training data, in the Initialize
initialize
method, make a history request.
training_length = 300 self.training_data = RollingWindow[float](training_length) history = self.history[TradeBar](self._symbol, training_length, Resolution.DAILY) for trade_bar in history: self.training_data.add(trade_bar.close)
Define a Training Method
To train the model, define a method that fits the model with the training data.
def get_features_and_labels(self, lookback=5): lookback_series = [] data = pd.Series(list(self.training_data)[::-1]) for i in range(1, lookback + 1): df = data.diff(i)[lookback:-1] df.name = f"close-{i}" lookback_series.append(df) X = pd.concat(lookback_series, axis=1).reset_index(drop=True).dropna() Y = data.diff(-1)[lookback:-1].reset_index(drop=True) return X.values, Y.values def my_training_method(self): features, labels = self.get_features_and_labels() # Define the loss function, we use MSE in this example def loss_mse(target_y, predicted_y): return tf.reduce_mean(tf.square(target_y - predicted_y)) # Train the model optimizer = tf.keras.optimizers.adam(learning_rate=self.learning_rate) for i in range(self.epochs): with tf.gradient_tape() as t: loss = loss_mse(labels, self.model(features)) jac = t.gradient(loss, self.model.trainable_weights) optimizer.apply_gradients(zip(jac, self.model.trainable_weights))
Set Training Schedule
To train the model at the beginning of your algorithm, in the Initialize
initialize
method, call the Train
train
method.
self.train(self.my_training_method)
To periodically re-train the model as your algorithm executes, in the Initialize
initialize
method, schedule some training sessions.
# Train the model every Sunday at 8:00 AM self.train(self.date_rules.every(DayOfWeek.SUNDAY), self.time_rules.at(8, 0), self.my_training_method)
Update Training Data
To update the training data as the algorithm executes, in the OnData
on_data
method, add the current close price to the RollingWindow
that holds the training data.
def on_data(self, slice: Slice) -> None: if self._symbol in slice.bars: self.training_data.add(slice.bars[self._symbol].close)
Predict Labels
To predict the labels of new data, in the OnData
on_data
method, get the most recent set of features and then call the run
method with new features.
new_features, __ = self.get_features_and_labels() prediction = self.model(new_features) prediction = float(prediction.numpy()[-1])
You can use the label prediction to place orders.
if prediction > 0: self.SetHoldings(self._symbol, 1) elif prediction < 0: self.SetHoldings(self._symbol, -1)
Save Models
Follow these steps to save Tensorflow
models into the Object Store:
- Set the key name of the model to be stored in the Object Store.
- Call the
GetFilePath
get_file_path
method with the key. - Call the
save
method with the model and file path. - Save the model to the file path.
model_key = "model.keras"
Note that the model has to have the suffix .keras
.
file_name = self.object_store.get_file_path(model_key)
This method returns the file path where the model will be stored.
model.save(file_name)
self.object_store.save(model_key)
Load Models
You can load and trade with pre-trained tensorflow
models that you saved in the Object Store. To load a tensorflow
model from the Object Store, in the Initialize
initialize
method, get the file path to the saved model and then recall the graph and weights of the model.
def initialize(self) -> None: model_key = 'model.keras' if self.object_store.contains_key(model_key): file_name = self.object_store.get_file_path(model_key) self.model = tf.keras.models.load_model(file_name)
The ContainsKey
contains_key
method returns a boolean that represents if the model.keras
is in the Object Store. If the Object Store doesn't contain the keys, save the model using them before you proceed.