Index Options
Universes
Create Subscriptions
Follow these steps to subscribe to an Index Option universe:
- Create a
QuantBook
. - Add the underlying Index.
- Call the
add_index_option
method with the underlyingIndex
Symbol
and, if you want non-standard Index Options, the target Option ticker.
qb = QuantBook()
index_symbol = qb.add_index("SPX", Resolution.MINUTE).symbol
To view the available Indices, see Supported Assets.
If you do not pass a resolution argument, Resolution.MINUTE
is used by default.
option = qb.add_index_option(index_symbol)
Price History
The contract filter determines which Index Option contracts are in your universe each trading day. The default filter selects the contracts with the following characteristics:
- Standard type (exclude weeklys)
- Within 1 strike price of the underlying asset price
- Expire within 35 days
To change the filter, call the set_filter
method.
# Set the contract filter to select contracts that have the strike price # within 1 strike level and expire within 90 days. option.set_filter(-1, 1, 0, 90)
To get the prices and volumes for all of the Index Option contracts that pass your filter during a specific period of time, call the option_history
method with the underlying Index Symbol
object, a start datetime
, and an end datetime
.
option_history = qb.option_history( index_symbol, datetime(2024, 1, 1), datetime(2024, 1, 5), Resolution.MINUTE, fill_forward=False, extended_market_hours=False )
To convert the OptionHistory
object to a DataFrame
that contains the trade and quote information of each contract and the underlying, use the data_frame
property.
option_history.data_frame
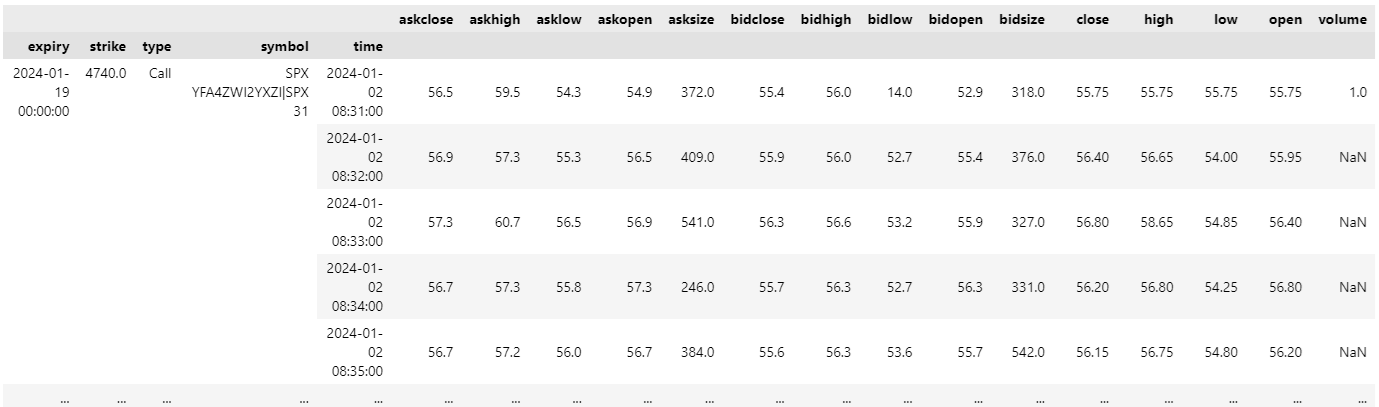
To get the expiration dates of all the contracts in an OptionHistory
object, call the method.
option_history.get_expiry_dates()
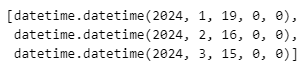
To get the strike prices of all the contracts in an OptionHistory
object, call the method.
option_history.get_strikes()
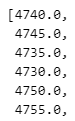
Daily Price and Greeks History
To get daily data on all the tradable contracts for a given date, call the history
method with the canoncial Option Symbol, a start date, and an end date. This method returns the entire Option chain for each trading day, not the subset of contracts that pass your universe filter. The daily Option chains contain the prices, volume, open interest, implied volaility, and Greeks of each contract.
# DataFrame format history_df = qb.history(option.symbol, datetime(2024, 1, 1), datetime(2024, 1, 5), flatten=True) # OptionUniverse objects history = qb.history[OptionUniverse](option.symbol, datetime(2024, 1, 1), datetime(2024, 1, 5)) for chain in history: end_time = chain.end_time filtered_chain = [contract for contract in chain if contract.greeks.delta > 0.3] for contract in filtered_chain: price = contract.price iv = contract.implied_volatility
The method represents each contract with an OptionUniverse
object, which have the following properties:
Examples
The following examples demonstrate some common practices for applying the Index Options dataset.
Example 1: Implied Volatility Line Chart
The following example plots a line chart on the implied volatility curve of the cloest expiring calls.
# Instantiate a QuantBook instance. qb = QuantBook() # Set the date being studied. date = datetime(2024, 1, 4) # Subscribe to the underlying Index. index_symbol = qb.add_index("SPX").symbol # Request the option data by calling the add_option method with the underlying Index Symbol. option = qb.add_option(index_symbol, "SPXW") option.set_filter(lambda u: u.include_weeklys()) # Request the historical option data to obtain the data to be calculated and compared. history_df = qb.history(option.symbol, date-timedelta(1), date, flatten=True).droplevel([0]) # Include only the closest expiring calls. expiry = min(x.id.date for x in history_df.index) history_df = history_df.loc[(x for x in history_df.index if x.id.date == expiry and x.id.option_right == OptionRight.CALL)] strikes = [x.id.strike_price for x in history_df.index] # Plot the IV curve versus strikes. history_df.index = strikes history_df[["impliedvolatility"]].plot(title="IV Curve", xlabel="strike", ylabel="implied volatility")
Example 2: COS Delta
The following example uses Fourier-Cosine method with finite differencing under Black-Scholes framework to calculate the option Delta. Then, we plot the actual Delta and the COS-calculated Delta to study the approximation accuracy. COS method can calculate a large number of option Greeks in a continuous strike range rapidly, making it suitable for arbitration and hedge position adjustment.
# Instantiate a QuantBook instance. qb = QuantBook() # Set the date being studied. date = datetime(2024, 1, 4) # Subscribe to the underlying Index. index_symbol = qb.add_index("SPX").symbol # Request the option data by calling the add_option method with the underlying Index Symbol. option = qb.add_option(index_symbol, "SPXW") option.set_filter(lambda u: u.include_weeklys()) # Request the historical option data to obtain the data to be calculated and compared. history_df = qb.history(option.symbol, date-timedelta(7), date, flatten=True).droplevel([0]) # Include only the furthest expiring puts. expiry = max(x.id.date for x in history_df.index) history_df = history_df.loc[(x for x in history_df.index if x.id.date == expiry and x.id.option_right == OptionRight.PUT)] strikes = np.array([x.id.strike_price for x in history_df.index]) # Get the implied volatility of the ATM put as the forward volatility forecast. history_df["underlying"] = history_df["underlying"].apply(lambda x: x.price) atm_call = sorted(history_df.iterrows(), key=lambda x: abs(x[0].id.strike_price - x[1].underlying))[0] iv = atm_call[1].impliedvolatility def psi_n(b1, b2, c, d, n): """Calculate the psi_n function used in the COS method.""" # Base case for n=0. if n == 0: return d - c delta_b = b2 - b1 # Compute sine terms for the given range. sin_terms = np.sin(n * np.pi * (np.array([d]) - b1) / delta_b) - np.sin(n * np.pi * (np.array([c]) - b1) / delta_b) return (delta_b * sin_terms) / (n * np.pi) def gamma_n(b1, b2, c, d, n): """Calculate the gamma_n function used in the COS method.""" base = n * np.pi / (b2 - b1) # Scaling factor based on n d_ = base * (d - b1) # Adjusted upper limit c_ = base * (c - b1) # Adjusted lower limit exp_d = np.exp(d) # Calculate exponential for d exp_c = np.exp(c) # Calculate exponential for c # Compute cosine and sine terms for the upper and lower limits. cos_terms = np.cos(np.array([d_, c_])) sin_terms = np.sin(np.array([d_, c_])) # Numerator of the gamma_n function. numerator = (cos_terms[0] * exp_d - cos_terms[1] * exp_c + base * (sin_terms[0] * exp_d - sin_terms[1] * exp_c)) return numerator / (1 + base**2) def v_n(b1, b2, c, d, n, K): """Calculate the v_n function, which combines psi_n and gamma_n.""" return 2 * K / (b2 - b1) * (psi_n(b1, b2, c, d, n) - gamma_n(b1, b2, c, d, n)) def log_char(t, S0, K, r, sigma, T): """Compute the characteristic function under BS model (log normal return)""" term1 = np.log(S0 / K) + (r - sigma**2 / 2) * T # Logarithmic term term2 = -sigma**2 * T * t**2 / 2 # Variance term return np.exp(1j * t * term1 + term2) def put(N, b1, b2, c, d, S0, K, r, sigma, T): """Calculate the price of a European put option using the COS method for multiple strike prices.""" price = np.zeros(len(K), dtype=np.complex_) # Calculate initial price for n=0. price += v_n(b1, b2, c, d, 0, K) * log_char(0, S0, K, r, sigma, T) / 2 # Loop over the series expansion for n from 1 to N. for i in range(1, N): t = i * np.pi / (b2 - b1) # Compute t for the current term log_char_terms = log_char(t, S0, K, r, sigma, T) # Evaluate characteristic function exp_term = np.exp(-1j * i * np.pi * b1 / (b2 - b1)) # Exponential decay term price += log_char_terms * exp_term * v_n(b1, b2, c, d, i, K) # Return the real part of the discounted price. return np.exp(-r * T) * price.real def option_delta(N, b1, b2, c, d, S0, K, r, sigma, T, delta_S=1e-5): """Calculate the delta of a European put option using finite differencing.""" # Calculate option price at the current asset price. price_current = put(N + 1, b1, b2, c, d, S0, K, r, sigma, T) # Calculate option price at slightly higher asset price. price_up = put(N + 1, b1, b2, c, d, S0 + delta_S, K, r, sigma, T) # Calculate delta by finite differencing. delta = (price_up - price_current) / delta_S return delta # Number of terms in the series expansion, the higher the more accurate. N = 50 # Underlying asset price S = atm_call[1].underlying # Number of fractional years till expiry. T = (expiry - date + timedelta(1)).total_seconds() / 60 / 60 / 24 / 365.25 # Get the interest rate and dividend yield to calculate the discount rate. r = np.log(1 + InterestRateProvider().get_interest_rate(date)) # continuous transformation q = DividendYieldProvider(index_symbol).get_dividend_yield(date) c1 = r - q # under risk-neutral measure c2 = T * iv**2 # Brownian variance c4 = 0 # Placeholder for additional variance (not used in this context) L = 10 # Parameter for the bounds # Calculate bounds for the COS method. b1 = c1 - L * np.sqrt(c2 + np.sqrt(c4)) # Lower bound b2 = c1 + L * np.sqrt(c2 + np.sqrt(c4)) # Upper bound # Calculate the put option price using the COS method. option_delta = option_delta(N + 1, b1, b2, b1, 0, S, strikes, r, iv, T) history_df.index = [x.id.strike_price for x in history_df.index] ax = history_df.delta.plot(title="Delta calculation using COS method", xlabel="strike", ylabel="delta", label="actual", legend=True) ax.plot(history_df.index, option_delta, label="COS") ax.legend()