Popular Libraries
Aesera
Get Historical Data
Get some historical market data to train and test the model. For example, to get data for the SPY ETF during 2020 and 2021, run:
qb = QuantBook() symbol = qb.add_equity("SPY", Resolution.DAILY).symbol history = qb.history(symbol, datetime(2020, 1, 1), datetime(2022, 1, 1)).loc[symbol]
Prepare Data
You need some historical data to prepare the data for the model. If you have historical data, manipulate it to train and test the model. In this example, use the following features and labels:
Data Category | Description |
---|---|
Features | Normalized close price of the SPY over the last 5 days |
Labels | Return direction of the SPY over the next day |
The following image shows the time difference between the features and labels:
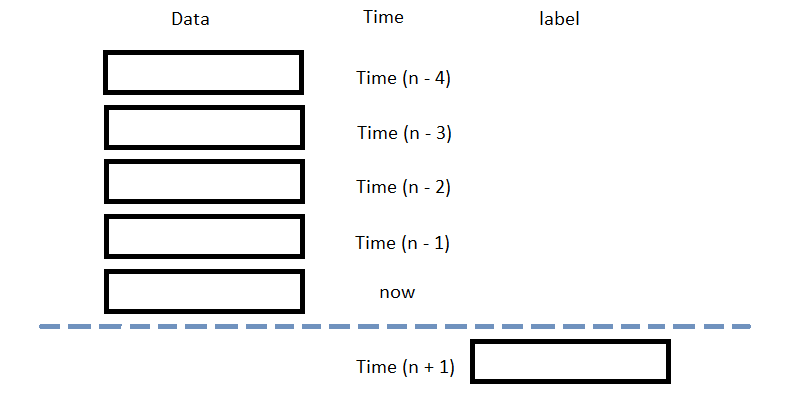
Follow these steps to prepare the data:
- Obtain the close price and return direction series.
- Loop through the
close
Series and collect the features. - Convert the lists of features and labels into
numpy
arrays. - Split the data into training and testing periods.
close = history['close'] returns = data['close'].pct_change().shift(-1)[lookback*2-1:-1].reset_index(drop=True) labels = pd.Series([1 if y > 0 else 0 for y in returns]) # binary class
lookback = 5 lookback_series = [] for i in range(1, lookback + 1): df = data['close'].shift(i)[lookback:-1] df.name = f"close-{i}" lookback_series.append(df) X = pd.concat(lookback_series, axis=1) # Normalize using the 5 day interval X = MinMaxScaler().fit_transform(X.T).T[4:]
X = np.array(features) y = np.array(labels)
X_train, X_test, y_train, y_test = train_test_split(X, y, test_size=0.2)
Train Models
You need to prepare the historical data for training before you train the model. If you have prepared the data, build and train the model. In this example, build a Logistic Regression model with log loss cross entropy and square error as cost function. Follow these steps to create the model:
- Generate a dataset.
- Initialize variables.
- Construct the model graph.
- Compile the model.
- Train the model with training dataset.
# D = (input_values, target_class) D = (np.array(X_train), np.array(y_train))
# Declare Aesara symbolic variables x = at.dmatrix("x") y = at.dvector("y") # initialize the weight vector w randomly using share so model coefficients keep their values # between training iterations (updates) rng = np.random.default_rng(100) w = aesara.shared(rng.standard_normal(X.shape[1]), name="w") # initialize the bias term b = aesara.shared(0., name="b")
# Construct Aesara expression graph p_1 = 1 / (1 + at.exp(-at.dot(x, w) - b)) # Logistic transformation prediction = p_1 > 0.5 # The prediction thresholded xent = y * at.log(p_1) - (1 - y) * at.log(1 - p_1) # Cross-entropy log-loss function cost = xent.mean() + 0.01 * (w ** 2).sum() # The cost to minimize (MSE) gw, gb = at.grad(cost, [w, b]) # Compute the gradient of the cost
train = aesara.function( inputs=[x, y], outputs=[prediction, xent], updates=((w, w - 0.1 * gw), (b, b - 0.1 * gb))) predict = aesara.function(inputs=[x], outputs=prediction)
pred, err = train(D[0], D[1]) # We can also inspect the final outcome print("Final model:") print(w.get_value()) print(b.get_value()) print("target values for D:") print(D[1]) print("prediction on D:") print(predict(D[0])) # whether > 0.5 or not
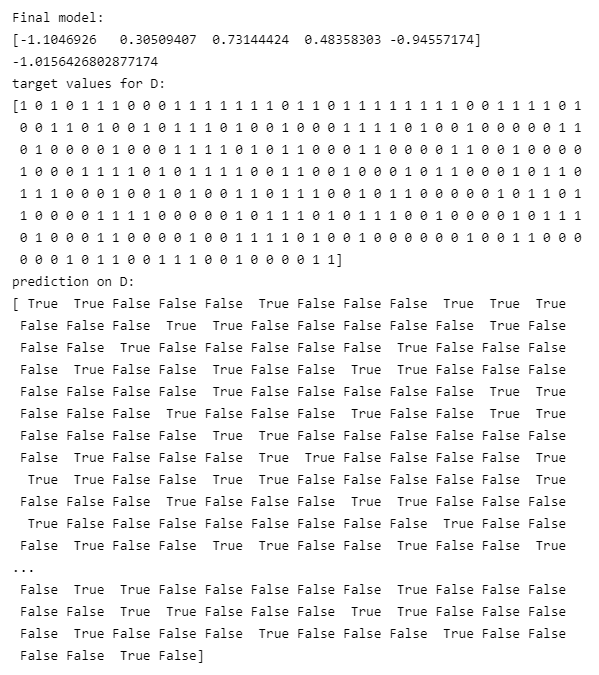
Test Models
You need to build and train the model before you test its performance. If you have trained the model, test it on the out-of-sample data. Follow these steps to test the model:
- Call the
predict
method with the features of the testing period. - Plot the actual and predicted labels of the testing period.
- Calculate the prediction accuracy.
y_hat = predict(np.array(X_test))
df = pd.DataFrame({'y': y_test, 'y_hat': y_hat}).astype(int) df.plot(title='Model Performance: predicted vs actual return direction in closing price', figsize=(12, 5))
correct = sum([1 if x==y else 0 for x, y in zip(y_test, y_hat)]) print(f"Accuracy: {correct}/{y_test.shape[0]} ({correct/y_test.shape[0]}%)")
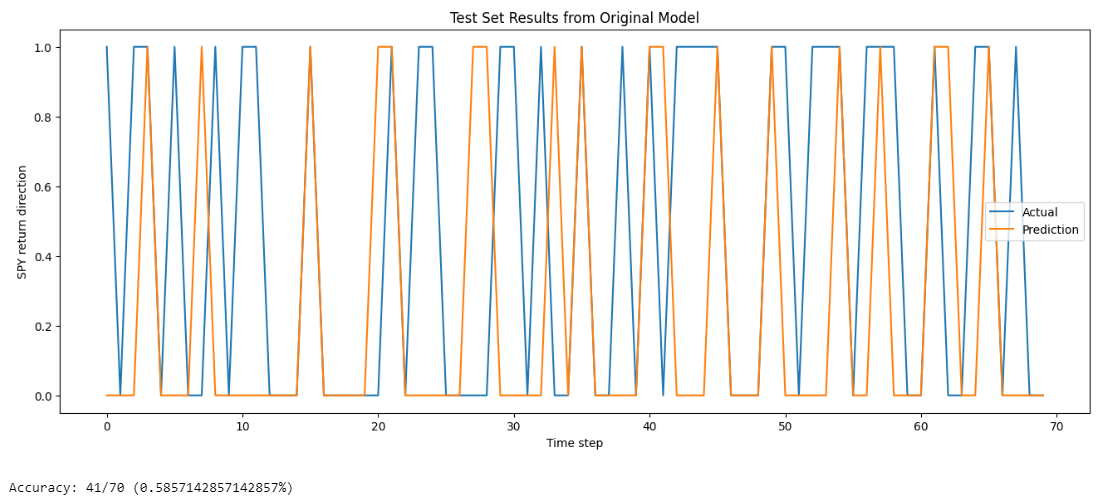
Store Models
You can save and load aesera
models using the Object Store.
Save Models
Follow these steps to save models in the Object Store:
- Set the key name of the model to be stored in the Object Store.
- Call the
GetFilePath
get_file_path
method with the key. - Call the
dump
method with the model and file path.
model_key = "model"
file_name = qb.object_store.get_file_path(model_key)
This method returns the file path where the model will be stored.
joblib.dump(predict, file_name)
If you dump the model using the joblib
module before you save the model, you don't need to retrain the model.
Load Models
You must save a model into the Object Store before you can load it from the Object Store. If you saved a model, follow these steps to load it:
- Call the
ContainsKey
contains_key
method with the model key. - Call
GetFilePath
with the key. - Call
load
with the file path.
qb.object_store.contains_key(model_key)
This method returns a boolean that represents if the model_key
is in the Object Store. If the Object Store does not contain the model_key
, save the model using the model_key
before you proceed.
file_name = qb.object_store.get_file_path(model_key)
This method returns the path where the model is stored.
loaded_model = joblib.load(file_name)
This method returns the saved model.
Examples
The following examples demonstrate some common practices for using the Aesera library.
Example 1: Predict Return Direction
The following research notebook uses Aesera
machine learning model to predict the next day's return direction by the previous 5 days' close price differences.
# Import the Aesera library and others. import aesara import aesara.tensor as at from sklearn.model_selection import train_test_split from sklearn.preprocessing import MinMaxScaler import joblib # Instantiate the QuantBook for researching. qb = QuantBook() # Request the daily SPY history with the date range to be studied. symbol = qb.add_equity("SPY", Resolution.DAILY).symbol history = qb.history(symbol, datetime(2020, 1, 1), datetime(2022, 1, 1)).loc[symbol] # We use the close price series to generate the features to be studied. close = history['close'] # Get the 1-day forward return direction as the labels for the machine to learn. returns = data['close'].pct_change().shift(-1)[lookback*2-1:-1].reset_index(drop=True) labels = pd.Series([1 if y > 0 else 0 for y in returns]) # binary class # Use 1- to 5-day differences as the input features for the machine to learn. lookback = 5 lookback_series = [] for i in range(1, lookback + 1): df = data['close'].shift(i)[lookback:-1] df.name = f"close-{i}" lookback_series.append(df) X = pd.concat(lookback_series, axis=1) # Normalize using the 5 day interval X = MinMaxScaler().fit_transform(X.T).T[4:] # Split the data as a training set and test set for validation. X = np.array(features) y = np.array(labels) X_train, X_test, y_train, y_test = train_test_split(X, y, test_size=0.2) # Create a dataset. D = (np.array(X_train), np.array(y_train)) # Declare Aesara symbolic variables. x = at.dmatrix("x") y = at.dvector("y") # Initialize the weight vector w randomly using share so model coefficients keep their values # between training iterations (updates). rng = np.random.default_rng(100) w = aesara.shared(rng.standard_normal(X.shape[1]), name="w") # initialize the bias term. b = aesara.shared(0., name="b") # Construct an Aesara expression graph. p_1 = 1 / (1 + at.exp(-at.dot(x, w) - b)) # Logistic transformation prediction = p_1 > 0.5 # The prediction thresholded xent = y * at.log(p_1) - (1 - y) * at.log(1 - p_1) # Cross-entropy log-loss function cost = xent.mean() + 0.01 * (w ** 2).sum() # The cost to minimize (MSE) gw, gb = at.grad(cost, [w, b]) # Compute the gradient of the cost # Compile the model. In this example, we set the step size as 0.1 times gradients. train = aesara.function( inputs=[x, y], outputs=[prediction, xent], updates=((w, w - 0.1 * gw), (b, b - 0.1 * gb))) predict = aesara.function(inputs=[x], outputs=prediction) # Train the model with the training dataset. pred, err = train(D[0], D[1]) # We can also inspect the final outcome print("Final model:") print(w.get_value()) print(b.get_value()) print("target values for D:") print(D[1]) print("prediction on D:") print(predict(D[0])) # whether > 0.5 or not # Predict the label of the testing set data. y_hat = predict(np.array(X_test)) # Plot and calculate the accuracy of the predicted testing set labels. df = pd.DataFrame({'y': y_test, 'y_hat': y_hat}).astype(int) df.plot(title='Model Performance: predicted vs actual return direction in closing price', figsize=(12, 5)) correct = sum([1 if x==y else 0 for x, y in zip(y_test, y_hat)]) print(f"Accuracy: {correct}/{y_test.shape[0]} ({correct/y_test.shape[0]}%)") # Store the model in the object store to allow accessing the model in the next research session or in the algorithm for trading. model_key = "model" file_name = qb.object_store.get_file_path(model_key) joblib.dump(predict, file_name)