Popular Libraries
Hmmlearn
Get Historical Data
Get some historical market data to train and test the model. For example, to get data for the SPY ETF during 2020 and 2021, run:
qb = QuantBook() symbol = qb.add_equity("SPY", Resolution.DAILY).symbol history = qb.history(symbol, datetime(2020, 1, 1), datetime(2022, 1, 1)).loc[symbol]
Prepare Data
You need some historical data to prepare the data for the model. If you have historical data, manipulate it to train and test the model. Follow these steps to prepare the data:
- Select the close column of the historical data DataFrame.
- Call the
pct_change
method and then drop the first row. - Call the
reshape
method.
closes = history['close']
daily_returns = closes.pct_change().iloc[1:]
X = daily_returns.values.reshape(-1, 1)
Train Models
You need to prepare the historical data for training before you train the model. If you have prepared the data, build and train the model. In this example, assume the market has only 2 regimes and the market returns follow a Gaussian distribution. Therefore, create a 2-component Hidden Markov Model with Gaussian emissions, which is equivalent to a Gaussian mixture model with 2 means. Follow these steps to create the model:
- Call the
GaussianHMM
constructor with the number of components, a covariance type, and the number of iterations. - Call the
fit
method with the training data.
model = hmm.GaussianHMM(n_components=2, covariance_type="full", n_iter=100)
model.fit(X)
Test Models
You need to build and train the model before you test its performance. If you have trained the model, test it on the out-of-sample data. Follow these steps to test the model:
- Call the predict method with the testing dataset.
- Plot the regimes in a scatter plot.
y = model.predict(X)
plt.figure(figsize=(15, 10)) plt.scatter(ret.index, [f'Regime {n+1}' for n in y]) plt.title(f'{symbol} market regime') plt.xlabel("time") plt.show()
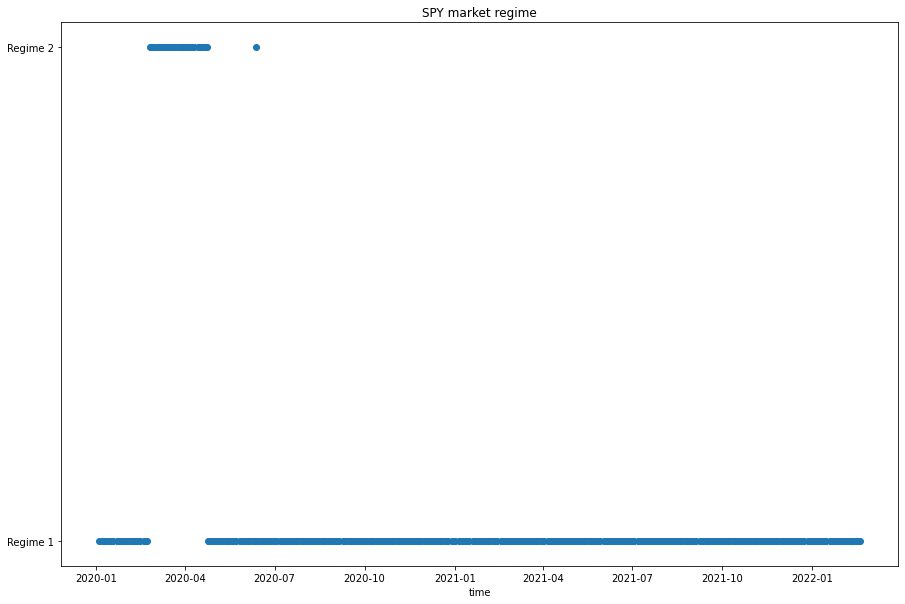
Store Models
You can save and load Hmmlearn
models using the Object Store.
Save Models
Follow these steps to save models in the Object Store:
- Set the key name of the model to be stored in the Object Store.
- Call the
GetFilePath
get_file_path
method with the key. - Call the
dump
method with the model and file path.
model_key = "model"
file_name = qb.object_store.get_file_path(model_key)
This method returns the file path where the model will be stored.
joblib.dump(model, file_name)
If you dump the model using the joblib
module before you save the model, you don't need to retrain the model.
Load Models
You must save a model into the Object Store before you can load it from the Object Store. If you saved a model, follow these steps to load it:
- Call the
ContainsKey
contains_key
method. - Call the
GetFilePath
get_file_path
method with the key. - Call the
load
method with the file path.
qb.object_store.contains_key(model_key)
This method returns a boolean that represents if the model_key
is in the Object Store. If the Object Store does not contain the model_key
, save the model using the model_key
before you proceed.
file_name = qb.object_store.get_file_path(model_key)
This method returns the path where the model is stored.
loaded_model = joblib.load(file_name)
This method returns the saved model.
Examples
The following examples demonstrate some common practices for using the Hmmlearn
library.
Example 1: Regime Detection
The following research notebook uses Hmmlearn
machine learning model to obtain the current market regime and predict the conditional probabilities of each market state that the current regime would turn into by a Hidden Markov Model (HMM).
# Import the Hmmlearn library and others. from hmmlearn import hmm import joblib # Instantiate the QuantBook for researching. qb = QuantBook() # Request the daily SPY history with the date range to be studied. symbol = qb.add_equity("SPY", Resolution.DAILY).symbol history = qb.history(symbol, datetime(2020, 1, 1), datetime(2022, 1, 1)).loc[symbol] # Obtain the daily returns to be analyzed. daily_returns = history['close'].pct_change()[1:] X = daily_returns.values.reshape(-1, 1) # Call the GaussianHMM constructor with the number of components, a covariance type, and the number of iterations to create the hidden markov model. model = hmm.GaussianHMM(n_components=2, covariance_type="full", n_iter=100) # Call the fit method with the training data to fit the model. model.fit(X) # Call the predict method with the testing dataset to get the prediction from the model. y = model.predict(X) # Plot the regimes in a scatter plot. plt.figure(figsize=(15, 10)) plt.scatter(ret.index, [f'Regime {n+1}' for n in y]) plt.title(f'{symbol} market regime') plt.xlabel("time") plt.show() # Store the model in the object store to allow accessing the model in the next research session or in the algorithm for trading. model_key = "model" file_name = qb.object_store.get_file_path(model_key) joblib.dump(model, file_name)