Futures Options
Individual Contracts
Introduction
This page explains how to request historical data for individual Future Option contracts. The history requests on this page only return the prices and open interest of the Option contracts, not their implied volatility or Greeks.
Create Subscriptions
Follow these steps to subscribe to individual Futures Option contracts:
- Load the assembly files and data types in their own cell.
- Import the data types.
- Create a
QuantBook
. - Add the underlying Futures contract.
- Set the start date to a date in the past that you want to use as the analysis date.
- Call the
OptionChain
option_chain
method with the underlying Futures contractSymbol
. - Sort and filter the data to select the specific Futures Options contract(s) you want to analyze.
- Call the
AddFutureOptionContract
add_future_option_contract
method with anOptionContract
Symbol and disable fill-forward.
#load "../Initialize.csx"
#load "../QuantConnect.csx" #r "../Microsoft.Data.Analysis.dll" using QuantConnect; using QuantConnect.Data; using QuantConnect.Algorithm; using QuantConnect.Research; using QuantConnect.Indicators; using QuantConnect.Data.Market; using QuantConnect.Securities; using Microsoft.Data.Analysis;
var qb = new QuantBook();
qb = QuantBook()
var future = qb.AddFuture(Futures.Indices.SP500EMini); var startDate = new DateTime(2023, 12, 20); var futuresContractSymbol = qb.FutureChainProvider.GetFutureContractList(future.Symbol, startDate) .OrderBy(s => s.ID.Date) .FirstOrDefault(); qb.AddFutureContract(futuresContractSymbol, fillForward: false);
future = qb.add_future(Futures.Indices.SP_500_E_MINI) start_date = datetime(2023, 12, 20) futures_contract_symbol = sorted( qb.future_chain_provider.get_future_contract_list(future.symbol, start_date), key=lambda s: s.id.date )[0] qb.add_future_contract(futures_contract_symbol, fill_forward=False)
To view the available underlying Futures in the US Future Options dataset, see Supported Assets.
qb.SetStartDate(futuresContractSymbol.ID.Date.AddDays(-5));
qb.set_start_date(futures_contract_symbol.id.date - timedelta(5))
The method that you call in the next step returns data on all the contracts that were tradable on this date.
var chain = qb.OptionChain(futuresContractSymbol);
chain = qb.option_chain(futures_contract_symbol, flatten=True).data_frame
This method returns an OptionChain
object, which represent an entire chain of Option contracts for a single underlying security.
You can even format the chain data into a DataFrame where each row in the DataFrame represents a single contract.
// Select a contract. var expiry = chain.Select(contract => contract.Expiry).Min(); var fopContractSymbol = chain .Where(contract => // Select call contracts with the closest expiry. contract.Expiry == expiry && contract.Right == OptionRight.Call ) // Select the contract with a strike price near the middle. .OrderBy(contract => contract.Strike) .ToList()[150] // Get the Symbol of the target contract. .Symbol;
# Select a contract. expiry = chain.expiry.min() fop_contract_symbol = chain[ # Select call contracts with the closest expiry. (chain.expiry == expiry) & (chain.right == OptionRight.CALL) # Select the contract with a strike price near the middle. ].sort_values('strike').index[150]
var optionContract = qb.AddFutureOptionContract(fopContractSymbol, fillForward: false);
option_contract = qb.add_future_option_contract(fop_contract_symbol, fill_forward=False)
Disable fill-forward because there are only a few OpenInterest
data points per day.
Trade History
TradeBar
objects are price bars that consolidate individual trades from the exchanges. They contain the open, high, low, close, and volume of trading activity over a period of time.
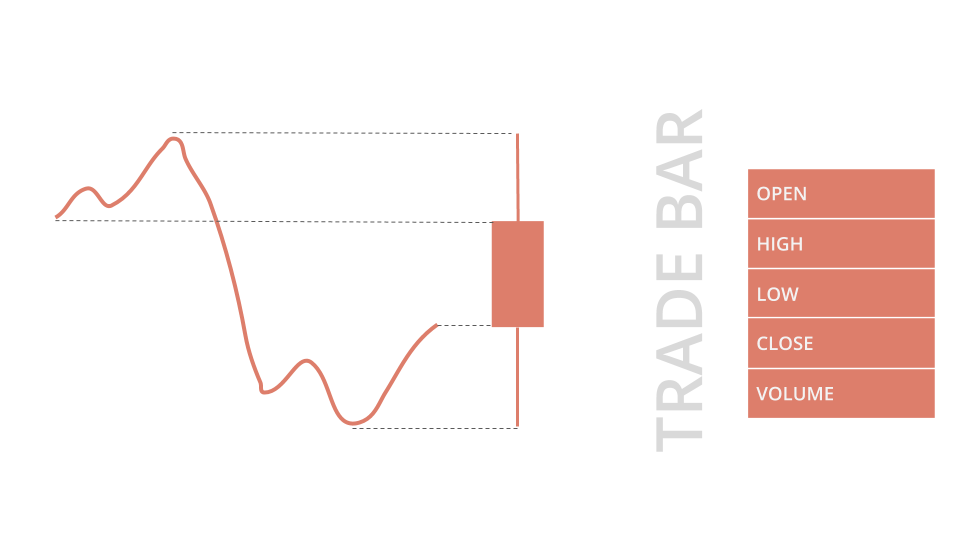
To get trade data, call the history
or history[TradeBar]
History<TradeBar>
method with the contract Symbol
object(s).
var history = qb.History<TradeBar>(fopContractSymbol, TimeSpan.FromDays(3)); foreach (var tradeBar in history) { Console.WriteLine(tradeBar); }
# DataFrame format history_df = qb.history(TradeBar, fop_contract_symbol, timedelta(3)) display(history_df) # TradeBar objects history = qb.history[TradeBar](fop_contract_symbol, timedelta(3)) for trade_bar in history: print(trade_bar)
TradeBar
objects have the following properties:
Quote History
QuoteBar
objects are bars that consolidate NBBO quotes from the exchanges. They contain the open, high, low, and close prices of the bid and ask. The Open
open
, High
high
, Low
low
, and Close
close
properties of the QuoteBar
object are the mean of the respective bid and ask prices. If the bid or ask portion of the QuoteBar
has no data, the Open
open
, High
high
, Low
low
, and Close
close
properties of the QuoteBar
copy the values of either the Bid
bid
or Ask
ask
instead of taking their mean.
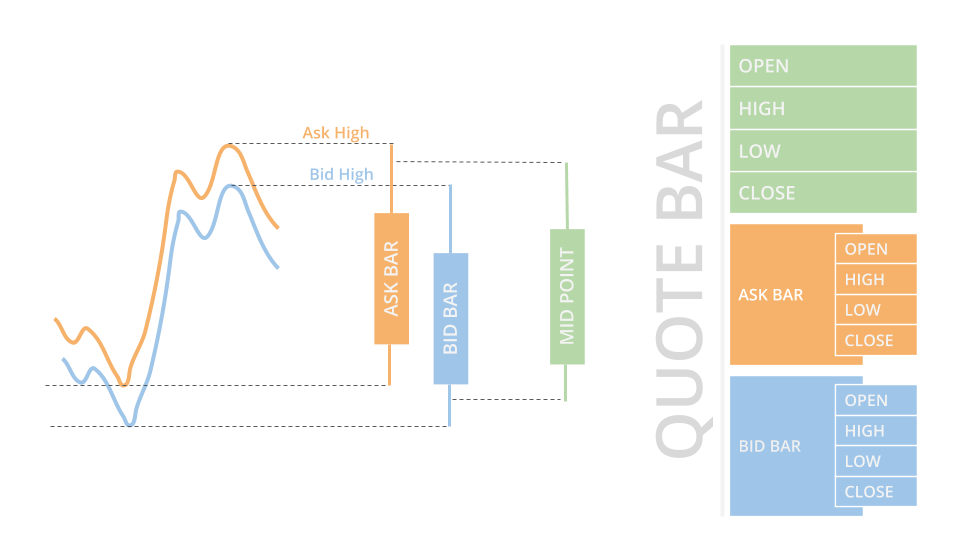
To get quote data, call the history
or history[QuoteBar]
History<QuoteBar>
method with the contract Symbol
object(s).
var history = qb.History<QuoteBar>(fopContractSymbol, TimeSpan.FromDays(3)); foreach (var quoteBar in history) { Console.WriteLine(quoteBar); }
# DataFrame format history_df = qb.history(QuoteBar, fop_contract_symbol, timedelta(3)) display(history_df) # QuoteBar objects history = qb.history[QuoteBar](fop_contract_symbol, timedelta(3)) for quote_bar in history: print(quote_bar)
QuoteBar
objects have the following properties:
Open Interest History
Open interest is the number of outstanding contracts that haven't been settled. It provides a measure of investor interest and the market liquidity, so it's a popular metric to use for contract selection. Open interest is calculated once per day.
To get open interest data, call the history
or history[OpenInterest]
History<OpenInterest>
method with the contract Symbol
object(s).
var history = qb.History<OpenInterest>(fopContractSymbol, TimeSpan.FromDays(3)); foreach (var openInterest in history) { Console.WriteLine(openInterest); }
# DataFrame format history_df = qb.history(OpenInterest, fop_contract_symbol, timedelta(3)) display(history_df) # OpenInterest objects history = qb.history[OpenInterest](fop_contract_symbol, timedelta(3)) for open_interest in history: print(open_interest)
OpenInterest
objects have the following properties:
Greeks and IV History
The Greeks are measures that describe the sensitivity of an Option's price to various factors like underlying price changes (Delta), time decay (Theta), volatility (Vega), and interest rates (Rho), while Implied Volatility (IV) represents the market's expectation of the underlying asset's volatility over the life of the Option.
Follow these steps to get the Greeks and IV data:
- Create the mirror contract
Symbol
. - Set up the risk free interest rate, dividend yield, and Option pricing models.
- Define a method to return the IV & Greeks indicator values for each contract.
- Call the preceding method and display the results.
var mirrorContractSymbol = Symbol.CreateOption( optionContract.Underlying.Symbol, fopContractSymbol.ID.Market, optionContract.Style, optionContract.Right == OptionRight.Put ? OptionRight.Call : OptionRight.Put, optionContract.StrikePrice, optionContract.Expiry );
mirror_contract_symbol = Symbol.create_option( option_contract.underlying.symbol, fop_contract_symbol.id.market, option_contract.style, OptionRight.Call if option_contract.right == OptionRight.PUT else OptionRight.PUT, option_contract.strike_price, option_contract.expiry )
In our research, we found the Forward Tree model to be the best pricing model for indicators.
risk_free_rate_model = qb.risk_free_interest_rate_model dividend_yield_model = DividendYieldProvider(futures_contract_symbol) option_model = OptionPricingModelType.FORWARD_TREE
var riskFreeRateModel = qb.RiskFreeInterestRateModel; var dividendYieldModel = new DividendYieldProvider(futuresContractSymbol); var optionModel = OptionPricingModelType.ForwardTree;
def greeks_and_iv(contracts, period, risk_free_rate_model, dividend_yield_model, option_model): # Get the call and put contract. call, put = sorted(contracts, key=lambda s: s.id.option_right) def get_values(indicator_class, contract, mirror_contract): return qb.indicator_history( indicator_class(contract, risk_free_rate_model, dividend_yield_model, mirror_contract, option_model), [contract, mirror_contract, contract.underlying], period ).data_frame.current return pd.DataFrame({ 'iv_call': get_values(ImpliedVolatility, call, put), 'iv_put': get_values(ImpliedVolatility, put, call), 'delta_call': get_values(Delta, call, put), 'delta_put': get_values(Delta, put, call), 'gamma_call': get_values(Gamma, call, put), 'gamma_put': get_values(Gamma, put, call), 'rho_call': get_values(Rho, call, put), 'rho_put': get_values(Rho, put, call), 'vega_call': get_values(Vega, call, put), 'vega_put': get_values(Vega, put, call), 'theta_call': get_values(Theta, call, put), 'theta_put': get_values(Theta, put, call), })
Dictionary<string, IndicatorHistory> GreeksAndIV(List<Symbol> contracts, int period) { // Get the call and put contract. var sortedSymbols = contracts.OrderBy(s => s.ID.OptionRight).ToArray(); var call = sortedSymbols[0]; var put = sortedSymbols[1]; IndicatorHistory GetValues(OptionIndicatorBase indicator) { // Use both contracts and the underlying to update the indicator and get its value. return qb.IndicatorHistory(indicator, new[] { call, put, call.Underlying }, period); } // Get the values of all the IV and Greek indicators. return new Dictionary<string, IndicatorHistory> { {"IVCall", GetValues(new ImpliedVolatility(call, riskFreeRateModel, dividendYieldModel, put, optionModel))}, {"IVPut", GetValues(new ImpliedVolatility(put, riskFreeRateModel, dividendYieldModel, call, optionModel))}, {"DeltaCall", GetValues(new Delta(call, riskFreeRateModel, dividendYieldModel, put, optionModel))}, {"DeltaPut", GetValues(new Delta(put, riskFreeRateModel, dividendYieldModel, call, optionModel))}, {"GammaCall", GetValues(new Gamma(call, riskFreeRateModel, dividendYieldModel, put, optionModel))}, {"GammaPut", GetValues(new Gamma(put, riskFreeRateModel, dividendYieldModel, call, optionModel))}, {"VegaCall", GetValues(new Vega(call, riskFreeRateModel, dividendYieldModel, put, optionModel))}, {"VegaPut", GetValues(new Vega(put, riskFreeRateModel, dividendYieldModel, call, optionModel))}, {"ThetaCall", GetValues(new Theta(call, riskFreeRateModel, dividendYieldModel, put, optionModel))}, {"ThetaPut", GetValues(new Theta(put, riskFreeRateModel, dividendYieldModel, call, optionModel))}, {"RhoCall", GetValues(new Rho(call, riskFreeRateModel, dividendYieldModel, put, optionModel))}, {"RhoPut", GetValues(new Rho(put, riskFreeRateModel, dividendYieldModel, call, optionModel))} }; }
greeks_and_iv([fop_contract_symbol, mirror_contract_symbol], 15, risk_free_rate_model, dividend_yield_model, option_model)
foreach (var (key, indicatorHistory) in GreeksAndIV(new List<Symbol> {fopContractSymbol, mirrorContractSymbol}, 15)) { foreach (var dataPoint in indicatorHistory) { Console.WriteLine($"{dataPoint.EndTime} - {key}: {dataPoint.Current.Value}"); } }
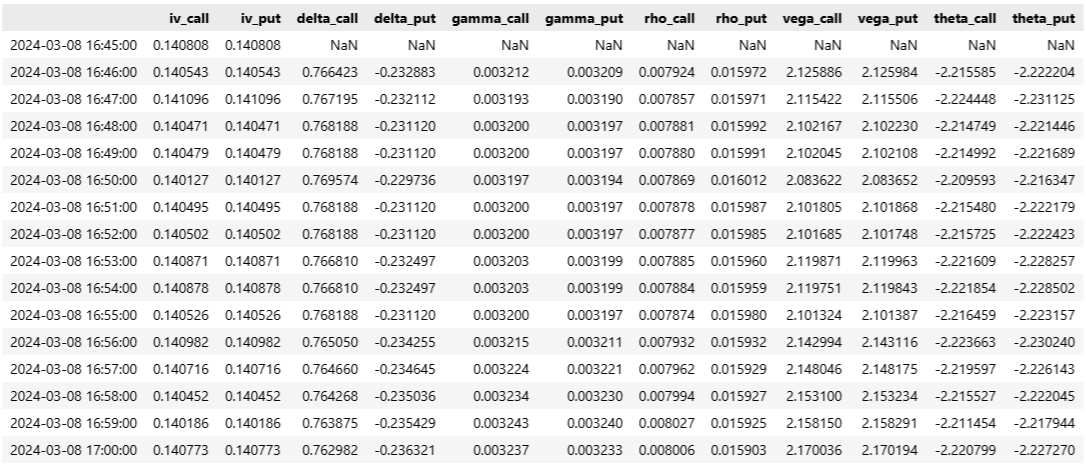
The DataFrame can have NaN entries if there is no data for the contracts or the underlying asset at a moment in time.
Examples
The following examples demonstrate some common practices for analyzing individual Future Option contracts in the Research Environment.
Example 1: Contract Mid-Price History
The following notebook plots the historical mid-prices of an E-mini S&P 500 Future Option contract using PlotlyPlotly.NET:
#load "../Initialize.csx"
import plotly.graph_objects as go # Add the underlying Future contract # (the front-month ES Future contract as of December 12, 2023). qb = QuantBook() future = qb.add_future(Futures.Indices.SP_500_E_MINI) futures_contract_symbol = sorted( qb.future_chain_provider.get_future_contract_list(future.symbol, datetime(2023, 12, 20)), key=lambda s: s.id.date )[0] qb.add_future_contract(futures_contract_symbol, fill_forward=False) # Get the Future Option chain as of 5 days before the underlying Future's expiry date. qb.set_start_date(futures_contract_symbol.id.date - timedelta(5)) chain = qb.option_chain(futures_contract_symbol, flatten=True).data_frame # Select a Future Option contract from the chain. expiry = chain.expiry.min() fop_contract_symbol = chain[ (chain.expiry == expiry) & (chain.right == OptionRight.CALL) ].sort_values('strike').index[50] # Add the target Future Option contract. qb.add_future_option_contract(fop_contract_symbol) # Get the Future Option contract quote history. history = qb.history(QuoteBar, fop_contract_symbol, datetime(2024, 2, 22), datetime(2024, 2, 23)) # Plot the mid-price values of the quote history. go.Figure( data=go.Candlestick( x=history.index.levels[4], open=history['open'], high=history['high'], low=history['low'], close=history['close'] ), layout=go.Layout( title=go.layout.Title(text=f'{fop_contract_symbol.value} OHLC'), xaxis_title='Date', yaxis_title='Price', xaxis_rangeslider_visible=False ) ).show()
#load "../QuantConnect.csx" #r "../Plotly.NET.dll" using QuantConnect; using QuantConnect.Data; using QuantConnect.Algorithm; using QuantConnect.Research; using QuantConnect.Indicators; using QuantConnect.Data.Market; using QuantConnect.Securities; using Plotly.NET; using Plotly.NET.LayoutObjects; // Add the underlying Future contract // (the front-month ES Future contract as of December 12, 2023). var qb = new QuantBook(); var future = qb.AddFuture(Futures.Indices.SP500EMini); var futuresContractSymbol = qb.FutureChainProvider .GetFutureContractList(future.Symbol, new DateTime(2023, 12, 20)) .OrderBy(s => s.ID.Date) .FirstOrDefault(); qb.AddFutureContract(futuresContractSymbol, fillForward: false); // Get the Future Option chain as of 5 days before the underlying Future's expiry date. qb.SetStartDate(futuresContractSymbol.ID.Date.AddDays(-5)); var chain = qb.OptionChain(futuresContractSymbol); // Select a Future Option contract from the chain. var expiry = chain.Select(contract => contract.Expiry).Min(); var fopContractSymbol = chain .Where(contract => contract.Expiry == expiry && contract.Right == OptionRight.Call) .OrderBy(contract => contract.Strike) .ToList()[50] .Symbol; // Add the target Future Option contract. qb.AddFutureOptionContract(fopContractSymbol); // Get the Future Option contract quote history. var history = qb.History<QuoteBar>(fopContractSymbol, new DateTime(2024, 2, 22), new DateTime(2024, 2, 23)); // Plot the mid-price values of the quote history. var chart = Chart2D.Chart.Candlestick<decimal, decimal, decimal, decimal, DateTime, string>( history.Select(x => x.Open), history.Select(x => x.High), history.Select(x => x.Low), history.Select(x => x.Close), history.Select(x => x.EndTime) ); LinearAxis xAxis = new LinearAxis(); xAxis.SetValue("title", "Time"); LinearAxis yAxis = new LinearAxis(); yAxis.SetValue("title", "Price ($)"); Title title = Title.init($"{fopContractSymbol} Price"); Layout layout = new Layout(); layout.SetValue("xaxis", xAxis); layout.SetValue("yaxis", yAxis); layout.SetValue("title", title); chart.WithLayout(layout); HTML(GenericChart.toChartHTML(chart))
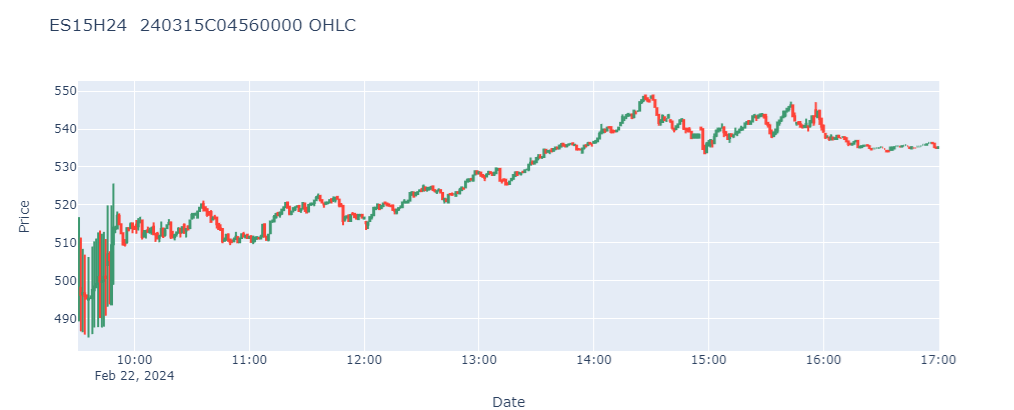
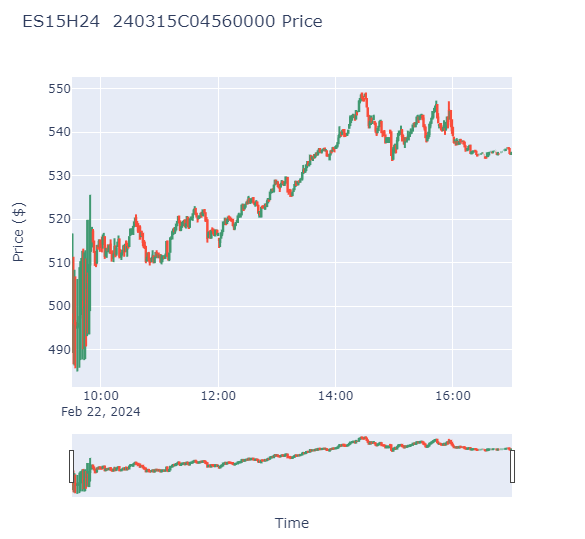