Introduction
In this research post, we examine a popular momentum strategy for intraday traders, the opening range breakout. To diversify our portfolio and reduce risk, we apply the strategy to a universe of liquid US Equities that are experiencing abnormally large trading volumes. The results show that the strategy outperforms buy and hold, achieving a 2.4 Sharpe ratio and a beta close to zero. The algorithm we implement in this post is a recreation of the research done by Zarattini, Barbon, & Aziz (2024).
Background
The opening range breakout strategy is a momentum strategy where we examine the asset's price action during the first n minutes of the day. If the price increases at the start of the day, we enter a long position when the price breaks above the highest price of the opening range. Conversely, if the price decreases during the start of the day, we enter a short position when the price breaks below the lowest price of the opening range. In this strategy, we use an opening range duration of 5 minutes.
Stocks in Play
We apply this strategy to a universe of assets to increase diversification and reduce risk. The universe consists of the 1,000 most liquid US Equities that are trading above $5/share and have an Average True Range (ATR) > $0.50. We then trade the 20 stocks that are most “in play,” meaning they have abnormally high trading volume, probably from some positive or negative catalyst. To quantify the stocks that are the most “in play,” we divide the asset’s volume during the first 5 minutes of trading activity in the current day by the average trading volume during the first 5 minutes of trading activity in the previous 14 days.
Risk Management
After one of the stocks in play breaks out of its opening range and we enter a position, there are two cases for exiting the position. In the first case, the momentum continues throughout the rest of the day, and we exit the position at close with a profit. In the second case, the stock reverts, hits our stop loss, and we exit the position before the market closes with a loss.
Stop Loss Placement
There are many techniques for placing a stop loss. In this strategy, we place the stop loss as a function of the entry price and the 14-day ATR. This technique means we apply wider stop losses to assets with greater volatility.
Position Sizing
The trade quantity is set so that if the stop loss is hit, we lose 1% of the portfolio value allocated to the asset. To reduce concentration risk, we limit the position size of each trade so that the weight of each asset doesn’t exceed the weight we would give the asset in an equal-weighted portfolio.
Implementation
To implement this strategy, we start by adding the universe of US Equities in the Initialize method.
_universe = AddUniverse(fundamentals => fundamentals
.Where(f => f.Price > 5 && f.Symbol != spy)
.OrderByDescending(f => f.DollarVolume)
.Take(_universeSize)
.Select(f => f.Symbol)
.ToList()
);
For each asset that enters the universe, we create a SymbolData object. At 9:35 AM, in the OnData method, we select the stocks in play and look for entries.
var filtered = ActiveSecurities.Values
.Where(s => s.Price != 0 && _universe.Selected.Contains(s.Symbol)).Select(s => _symbolDataBySymbol[s.Symbol]).Where(s => s.RelativeVolume > 1 && s.ATR > _atrThreshold)
.OrderByDescending(s => s.RelativeVolume).Take(MaxPositions);
foreach (var symbolData in filtered)
{
symbolData.Scan();
}
The Scan method uses the opening range bar to determine the stop price for the entry and exit orders.
if (OpeningBar.Close > OpeningBar.Open)
{
PlaceTrade(OpeningBar.High, OpeningBar.High - _stopLossAtrDistance * ATR);
}
else if (OpeningBar.Close < OpeningBar.Open)
{
PlaceTrade(OpeningBar.Low, OpeningBar.Low + _stopLossAtrDistance * ATR);
}
The PlaceTrade method determines the trade size, places the entry order, and records the stop loss price.
var quantity = (int)((_stopLossRiskSize * _algorithm.Portfolio.TotalPortfolioValue / _algorithm.MaxPositions) / (entryPrice - stopPrice));
var quantityLimit = _algorithm.CalculateOrderQuantity(_security.Symbol, 1m/_algorithm.MaxPositions);
quantity = (int)(Math.Min(Math.Abs(quantity), quantityLimit) * Math.Sign(quantity));
if (quantity != 0)
{
StopLossPrice = stopPrice;
EntryTicket = _algorithm.StopMarketOrder(_security.Symbol, quantity, entryPrice, $"Entry");
}
Results
We backtested the algorithm during 2016, the first year of the paper's backtest period. The benchmark is buy-and-hold with the SPY, which produced a 0.836 Sharpe ratio. In contrast, the opening range breakout strategy generated a 2.396 Sharpe ratio and a -0.042 beta. Therefore, the strategy outperformed buy-and-hold.
To test the sensitivity of the parameters chosen, we ran a parameter optimization job. We tested opening range durations of 5 to 25 minutes in steps of 5 minutes, and we tested universe sizes of 500 to 1500 US Equities in steps of 250. Of the 25 parameter combinations, 17 (68%) produced a greater Sharpe ratio than the benchmark. The following image shows the heatmap of Sharpe ratios for the parameter combinations:
The red circle in the preceding image identifies the parameters we chose as the default parameter for the strategy. We chose an opening range duration of 5 minutes because it was the best-performing duration of all the intervals tested by Zarattini et al. (2024). We chose a universe size of 1,000 because it was a round number that was large enough to diversify the strategy across many assets yet small enough that the backtest could run in under 10 minutes.
All of the parameters in this implementation match the parameters selected by the original authors. The only exception is the size of the universe. Zarattini et al. (2024) use a universe of 7,000 US Equities. However, the preceding optimization result shows that any universe size we selected produces a Sharpe ratio above 2, outperforming the benchmark.
It seemed odd to filter the ATR according to an arbitrary absolute value when the price of the stocks in the portfolio can be at greatly different scales. To ensure this parameter ($0.50) was not a cherry-picked value, we ran an optimization to test the sensitivity of the strategy to the ATR value. We tested $0 ATR to $2 ATR in steps of $0.25. We discovered all of these ATR values outperformed buy-and-hold, with Sharpe ratios ranging from 1.5 to 2.7.
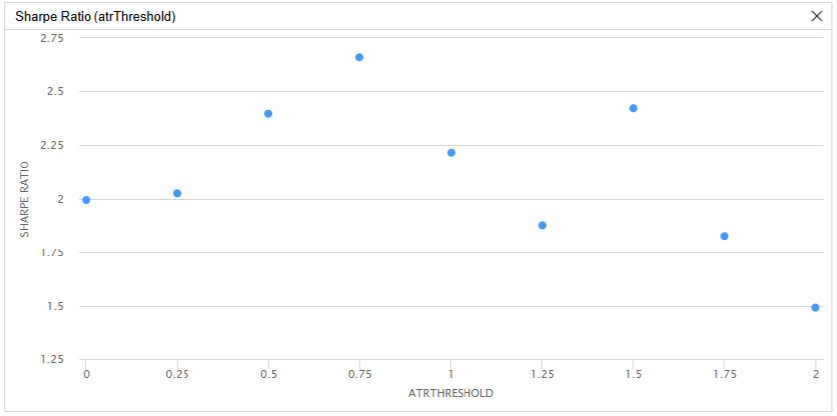
In addition to testing the sensitivity of ATR dollar values, we adjusted the filter to select stocks that had an ATR above 1% of the asset's price, effectively making the filter unit-less. With this adjustment, the algorithm still produced a 2.237 Sharpe ratio and a 97% Probabilistic Sharpe Ratio.
References
- Zarattini, Carlo and Barbon, Andrea and Aziz, Andrew, A Profitable Day Trading Strategy For The U.S. Equity Market (February 16, 2024). Swiss Finance Institute Research Paper No. 24-98, Available at SSRN: https://ssrn.com/abstract=4729284 or http://dx.doi.org/10.2139/ssrn.4729284
Sylvain Thibault
Great post, would be great to have the equivalent Python code.
Petr Zurek
How does it perform from 2016 - 2024. Oh wait, I can test it myself ;-)
Derek Melchin
Here is a Python implementation of the strategy. It's not exactly the same as the C# version above, so expect slightly different results. For instance, this version doesn't have the ATR filter and it relies on both daily and minute resolution data subscriptions, so indicators may not be accurate. For the most accurate results and execution speed, use the C# version above.
The material on this website is provided for informational purposes only and does not constitute an offer to sell, a solicitation to buy, or a recommendation or endorsement for any security or strategy, nor does it constitute an offer to provide investment advisory services by QuantConnect. In addition, the material offers no opinion with respect to the suitability of any security or specific investment. QuantConnect makes no guarantees as to the accuracy or completeness of the views expressed in the website. The views are subject to change, and may have become unreliable for various reasons, including changes in market conditions or economic circumstances. All investments involve risk, including loss of principal. You should consult with an investment professional before making any investment decisions.
Enjolras Leigh
The backtest doesn't look good for other years. Does it overfit?
Why so many KOL are teaching this pattern? did they do the backtest?
AleNoc
Thank's for the post, I was just trying to implement this strategy it in these weeks :) ; any further suggestions regarding order execution with possible slippage in real trading?
Jack Pizza
thing is how to get these trading costs under control…. 25% that's only trading 6 symbols….
You can argue the fees are covered if IBKR gives you price improvements, or can use a free broker that will probably get you worst fills… so you end up in the same boat.
Yuri Lopukhov
This is my attempt at replicating it in Python, it is close, but not as good. Upon closer look, ATR in Python are 3x lower or so, also, on first entry ATR has ~7k samples, this looks insane for daily indicator. In C# code it has only 33 samples, which looks correct to me. It looks like for some reason it is being warmed up with minute resolution bars instead of daily. This looks similar to this bug I necountered before: IdentityDataConsolidator Does Not Handle Fill Forward Correctly · Issue #8392 · QuantConnect/Lean Or maybe I did something wrong…
Because of this, stop loss is too close and triggers far too often. Workaround shouldn't be too difficult, I just won't work on it today…
Backtesting time of Python version is 1588.03 seconds vs 730.75 seconds of C# version on B2-8 node. Definitely use C# for optimization or backtesting on larger date spans.
Overall, strategy looks good to me as long as the market is not crashing. Maybe add some filters to detect that. It has low win rate, but high profit ratio to accomodate for that. Stop loss triggers for most of the trades. Perhaps there is room to improvement in symbols selection, entry orders or risk management…
Yuri Lopukhov
I actually found a mistake in my code, here is a fixed version. It's almost a match with C# version, except its a little bit better )))
It also took 1171.79 seconds vs 1588.03 from the previous Python version…
.ekz.
Great to see this! i actually worked on this for python some months ago but it didnt seem to work too well on a single symbol so I doubted their reserarch was credible. Now i see i was wrong and i should i have tried harder :)
My goal was to create a system that uses the proven ORB entry, but trades options instead, based on IV Rank and Gamma exposure. I believe there is some alpha there.
Now i can do actually give my goal another shot. Thank you Derek Melchin and Yuri Lopukhov .
Including a link to my technical spec if anyone wants to give feedback or try building it as well!
The GEX-Enhanced Opening Range Options Breakout Strategy
( Sorry I tried to delete previous post where i pasted the whole thing, but I was too late. someone from QC team please help cc: Derek Melchin )
Jack Pizza
@yuri_lopukhov
I already tried with momentum filters, problem is the returns are just too painfully low already... adding that filter made them go down even more... as it would sit out of market. Also tried adding them individually to only trade long with positive momentum and only trade short with negative momentum same thing.
next step is maybe trying an MA filter... but suspect same results...
I tried hard coding MEGA stocks META, NVDA Ect. completely crashes in 08'
Also noticed if you raise the filter to stocks > $50 performance goes down the can too, so it seems like there might be some sort of bias because out of a universe of 1000 stocks there is plenty to choose from, so starting to think performance has more to do with the actual selected stock vs all the ATR selection process.
Jack Pizza
or i guess ATR makes sense with smaller stocks, as if it increases that much, it means some major news has happened and it will effect it more profoundly than say a mega cap… not sure.
Jack Pizza
Also completely collapses during dot com, for some reason it does decent in 07-08, and only around a 10% DD during covid…
Mark Kust
Has anyone tried the contrarian trade? For example, when a stock is identified as having a long opening range breakout, flag it for shorting once it reverses below that same level. Vice versa for short opening range breakouts. The fact that the algorithm as written has a 17% win rate (83% loss rate), kind of makes this the obvious follow-up. Would then set the stop-out level to 1 ATR above the entry (and vice-versa for shorts). If no one has tried this yet, I may go ahead and implement it. Obviously, I don't know that this will work, but can't help thinking that I'd like to see it.
AleNoc
Which is the minimum RAM needed to deploy live this code? It could be nice to implement some control also to check if orders became invalid and not accepted by the broker, for example Alpaca in paper trading rejected me some stop orders as “Wash trade” invalidating the strategy
Yuri Lopukhov
Just a heads up: this condition is really not working well on resolution lower than minute and most likely in live deployments:
Basically, on every new piece of data in this minute it will place entry orders. On live instance I believe it will run for every new group of ticks…
Easiest fix would be to add a variable to check if entries were already placed today and reset it at the end of the day or on the beginning. I might share updated C# code a bit later (not really working with Python version since C# is faster and most likely requires less RAM, which is important for live instance…)
Yuri Lopukhov
Here is an updated C# version, also added some extra features and plots
Projectedxyz
Given the fees, this strategy seems tailor made for TradeStation $0 commissions for equities, no?
Yuri Lopukhov
Ok, so I've identified an issue with transferring backtesting results to live trading:
We place stop loss order after entry stop order is filled. In backtesting (or live trading with QC paper account) with minute resolution this means that stop order will be placed on the next minute after entry order is filled. In real-time or on lower resolution, e.g. second/tick, stop order will be placed as soon as entry was filled, so it can be on the next tick or second.
So for example, entry order may be filled at 9:35:01, and in real-time stop order will be placed at that time, while in backtesting it will be placed at 9:36:00. This creates quite a difference between backtesting and live trading.
There are two solution I can see here:
Any other thoughts?
P.S. I was able to run C# version on lowest tier node with 500 securities in universe with minute resolution and with 250 securities on second resolution. It may run out of RAM during warm up, but on the next attempt it usually succeeds.
P.P.S. if anyone feels risky, they can unleash full leverage by changing 1m to something up to 4m in this line:
AleNoc
In the backtest we could use something like this in public override void Initialize() to boost performance with seconds resolution:
Lars Klawitter
Hi all,
Happy new year and many thanks to Derek and Yuri for the great work!
Having played with Yuri's implementation (both the python version and also the most recent C# version for performance reasons), I noticed that the risk adjusted returns increase significantly with larger universe sizes and with shorter opening ranges.
A universe of 2000 and openingRangeMinutes as short as 1 minute does really well:
This was based on a 2020-2022 three year backtest, but the above pattern (larger universeSizes and openingRangeMinutes of 1 or 2 minute doing significantly better than longer ranges and smaller universes) is consistent over all backtest periods between 2010 and today that I tried.
An L2-4 node will happily run a 2000 Universe.
The most significant improvement though, comes from the shortening of the openingRange to just one minute, which would make sense considering that both algorithmic trading and MOO orders would cause a lot of the breakout momentum described in the paper within the first minute after market open.
I'm paper trading with this set of parameters right now, as I'm not sure if this assumption holds up in reality…
Derek Melchin
The material on this website is provided for informational purposes only and does not constitute an offer to sell, a solicitation to buy, or a recommendation or endorsement for any security or strategy, nor does it constitute an offer to provide investment advisory services by QuantConnect. In addition, the material offers no opinion with respect to the suitability of any security or specific investment. QuantConnect makes no guarantees as to the accuracy or completeness of the views expressed in the website. The views are subject to change, and may have become unreliable for various reasons, including changes in market conditions or economic circumstances. All investments involve risk, including loss of principal. You should consult with an investment professional before making any investment decisions.
To unlock posting to the community forums please complete at least 30% of Boot Camp.
You can continue your Boot Camp training progress from the terminal. We hope to see you in the community soon!