About US Equities
The US Equities dataset by AlgoSeek is survivorship bias-free daily coverage of every stock traded in the US Securities Information Processors (SIP) CTA/UTP feed since 1998. The dataset covers approximately 27,500 securities, starts in January 1998, and is delivered in any resolution from tick to daily. The Data is collected from the full SIP feed via our Equinix co-located servers, including all trades and quotes published to every exchange as well as FINRA. Over-the-Counter (OTC) trades are not included.
This dataset depends on the US Equity Security Master dataset because the US Equity Security Master dataset contains information on splits, dividends, and symbol changes.
About AlgoSeek
AlgoSeek is a leading historical intraday US market data provider offering the most comprehensive and detailed market data and analytics products in the financial industry covering Equities, Futures, Options, cash FOREX, and Cryptocurrencies. AlgoSeek data is built for quantitative trading and machine learning. For more information about AlgoSeek, visit algoseek.com.
About QuantConnect
QuantConnect was founded in 2012 to serve quants everywhere with the best possible algorithmic trading technology. Seeking to disrupt a notoriously closed-source industry, QuantConnect takes a radically open-source approach to algorithmic trading. Through the QuantConnect web platform, more than 50,000 quants are served every month.
Algorithm Example
from AlgorithmImports import *
from QuantConnect.DataSource import *
class USEquityDataAlgorithm(QCAlgorithm):
def initialize(self) -> None:
self.set_start_date(2018, 1, 1)
self.set_end_date(2021, 6, 1)
self.set_cash(100000)
# Requesting single equity data, since we only trade AAPL
self.aapl = self.add_equity("AAPL", Resolution.DAILY).symbol
# Historical data
history = self.history(self.aapl, 60, Resolution.DAILY)
self.debug(f"We got {len(history)} items from our history request")
def on_data(self, slice: Slice) -> None:
# Check if the current slice containing AAPL and if we hold any position
# As we make use of the most updated price data to decide the order size
if slice.contains_key(self.aapl) and slice[self.aapl] is not None and not self.portfolio.invested:
self.set_holdings(self.aapl, 1)
Example Applications
The US Equities dataset enables you to accurately design Equity trading strategies. Examples include the following strategies:
- Momentum strategies using historical returns on the premise that the momentum will continue
- Value strategies using fundamental factors on the premise that the price of undervalued securities will rise
- Factor investing with periodic rebalancing
Pricing
Cloud Access
Freely harness terabytes of US Equities data in the QuantConnect Cloud for your backtesting and live trading purposes.
Tick Download
US Equity Tick resolution archives in LEAN format for on premise backtesting and research. One file per ticker/day.
Second Download
US Equity Second resolution archives in LEAN format for on premise backtesting and research. One file per ticker/day.
Minute Download
US Equity Minute resolution archives in LEAN format for on premise backtesting and research. One file per ticker/day.
Hour Download
US Equity Hourly resolution archives in LEAN format for on premise backtesting and research. One file per ticker.
Daily Download
US Equity Daily resolution archives in LEAN format for on premise backtesting and research. One file per ticker.
Bulk Minute Updates
Bulk download minute data
Bulk Second Updates
Bulk download second data
Bulk Tick Updates
Bulk download tick data
Bulk Hour Updates
Bulk download hourly data
Bulk Daily Updates
Bulk download of daily resolution data
Bulk Hour Download
Bulk download hourly data
Bulk Daily Download
Bulk download of daily resolution data
Bulk Minute Download
Bulk download minute data
Bulk Second Download
Bulk download second data
Bulk Tick Download
Bulk download tick data
Explore Other Datasets
US Equity Coarse Universe
Dataset by QuantConnect
US Futures
Dataset by AlgoSeek
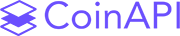
Bybit Crypto Price Data
Dataset by CoinAPI